17th International Conference on Cytopathology and Histopathology
Vancouver, Canada
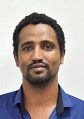
Jacob Amsalu Bayisa
National Taiwan University of Science and Technology, Taiwan
Title: Automatic cervical pre-cancerous cell detection from pap smear samples using deep learning
Biography
Biography: Jacob Amsalu Bayisa
Abstract
According to the 2018 WHO cancer report, cervical cancer is the fourth commonest type of cancer in women. For early screening of cervical cancer pap test and liquid cytology are commonly used, and diagnosis of pre-cancerous cells heavily relies heavily on the experience of the clinician for an accurate diagnosis. The collected cells are manually scanned under the microscope by a pathologist. Especially in developing countries, there is a shortage of clinicians and screening service is largely unavailable. Nowadays a subtype of machine learning called deep learning technologies is heavily being implemented for various clinical purposes. We developed an automatic cervical pre-cancerous cell detection technique using a deep learning model, that can be used for screening of cervical cancer. For training of our model, we used pap smear picture data which was collected from the DTU/Herlev Pap Smear Databases which contains 917 pap smear images. We used 80% of data for training and 10% for validation and 10% for tests. We also used the data augmentation method using the generative adversarial network to enhance model generalization. Our deep learning model is similar to U-Net structure and was able to detect precancerous cell with an accuracy of 94% which is a state of the art performance and can be used for automatic screening of cervical cancer. The trained model can also be easily deployed to a portable device like mobile phones and tablets.